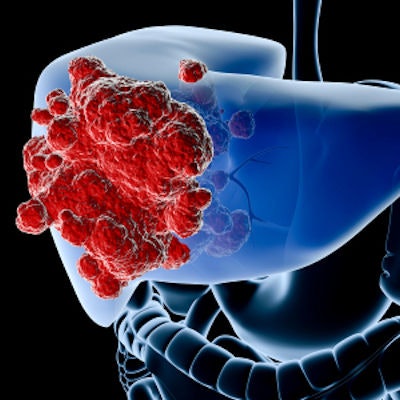
With help from AI and radiomics analysis, dual-energy CT (DECT) can be an effective way to diagnose an aggressive macrotrabecular-massive subtype of hepatocellular carcinoma (HCC), a research team has found.
"[Our study showed that] a deep-learning radiomics nomogram derived from multiparametric DECT accurately predicted the [macrotrabecular-massive] subtype in patients with HCC," wrote a team led by Mengsi Li, PhD, of Sun Yat-Sen University in Guangdong, China. The group's findings were published August 22 in Radiology.
Macrotrabecular-massive HCC is an aggressive variant of the disease that the World Health Organization identified in 2019, according to the investigators. It tends to be diagnosed via preoperative biopsy or postoperative histopathology, but noninvasive alternatives are needed, they noted.
Dual-energy CT is a relatively new CT technology that can characterize tissue beyond conventional CT, and combining radiomic features from DECT exams with deep-learning algorithms shows promise for the diagnosis of rare HCC subtypes, according to Li and colleagues.
To assess DECT's performance for this indication, the team conducted a study that included 262 patients with histopathologically confirmed HCC who underwent contrast-enhanced DECT between June 2019 and June 2022. The patients were recruited from three hospitals and divided into two different data sets for the deep-learning model the team developed; patients from center 1 were included in a training set (n = 146) and an internal data set (n = 35), and those from centers 2 and 3 in an external test data set (n = 81). The investigators compared the deep-learning model's performance to a clinical-radiologic model using the area under the receiver operating characteristic curve (AUC) measure.
The deep-learning model better predicted the presence of the aggressive macrotrabecular-massive subtype of HCC than did the clinical-radiologic model, the group found. Its sensitivity across all data sets was comparable and its specificity was especially higher in the external test set.
Performance of clinical-radiologic model versus deep-learning model for diagnosing HCC | ||
Measure | Clinical-radiologic model | Deep-learning radiomics nomogram |
AUC | ||
Training data set | 0.77 | 0.91 |
Internal test set | 0.72 | 0.87 |
External test set | 0.79 | 0.89 |
Sensitivity | ||
Training data set | 82% | 95% |
Internal test set | 90% | 80% |
External test set | 87% | 80% |
Specificity | ||
Training data set | 58% | 77% |
Internal test set | 56% | 72% |
External test set | 63% | 90% |
The deep-learning model offers clinicians a better way to identify liver cancer subtypes such as macrotrabecular-massive HCC, according to a commentary that accompanied the study.
"The current literature suggests that radiomics and [deep learning] can improve our ability to noninvasively predict the aggressive [macrotrabecular-massive] phenotype and to identify the patients most likely to benefit from neoadjuvant therapy," wrote Linda Chu, MD, and colleague Elliot Fishman, MD, both of Johns Hopkins in Baltimore. "Ideally, there will be parallel therapeutic advances that target the underlying molecular and pathologic alterations to maximize the impact of accurate preoperative HCC phenotyping."
The complete study can be found here.