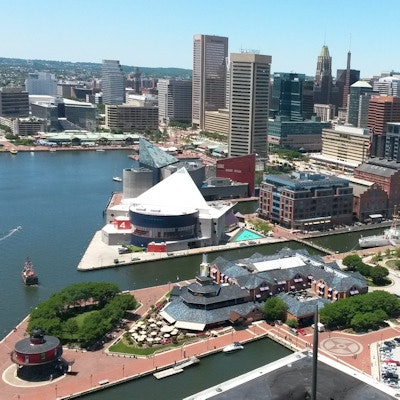
BALTIMORE - A recent U.S. Food and Drug Administration (FDA) ruling will make it easier to commercialize artificial intelligence (AI) software for medical imaging applications, an FDA representative said at the Society for Imaging Informatics in Medicine's Conference on Machine Intelligence in Medical Imaging (C-MIMI).
The FDA issued a decision in July that classified a breast imaging computer-aided diagnosis (CADx) software with AI technology as a class II device and established a new generic product type. As a result, vendors with similar products can now apply for 510(k) clearance instead of having to go through the more rigorous premarket approval (PMA) process used for class III devices, according to Berkman Sahiner, PhD, leader of the FDA's Division of Imaging, Diagnostics, and Software Reliability (DIDSR) Image Analysis Laboratory.
Sahiner discussed the significance of that ruling, as well as other relevant FDA initiatives and perspectives on machine learning and software development for medical image interpretation, during a keynote talk Tuesday morning at C-MIMI 2017.
FDA submission types
In general terms, the FDA regulates medical devices based on their risk to patients and their intended use. Devices are categorized into one of three classes: class I (low-risk devices such as medical gloves, class II (such as CT scanners), or class III (the highest-risk devices such as stents). The most common regulatory path to market for medical devices is the 510(k) premarket notification process, which requires demonstration that a new device is substantially equivalent to a legally marketed device -- i.e., a predicate device, Sahiner said.
The premarket approval (PMA) process is required for class III devices, and companies must demonstrate a reasonable assurance of safety and effectiveness of these devices. Vendors can also request a de novo classification for novel devices that have not been previously classified by the FDA and are therefore deemed to be class III by default. The de novo petition requests that a product be down-classified (from class III to class II or class II to class I). These petitions must propose controls that would be needed to ensure the safety and effectiveness of the device, according to Sahiner.
Importantly, a granted de novo classification establishes a new device type, a new device classification, and a new regulation, as well as necessary general (and special) controls, Sahiner said.
"Once the de novo application is granted, this device is eligible to serve as a predicate for 510(k) devices that have similar intended risk," he said. "All the followers are 510(k) devices."
Sahiner also recommends that companies developing new software contact the FDA for information and consultation on their device to avoid delays in a formal submission or having to repeat clinical studies.
CADe and CADx
Machine-learning algorithms in image interpretation have two applications: computer-aided detection (CADe) and CADx. CADe is used for detecting abnormalities and can be used as a first reader or sequentially or concurrently by users, Sahiner said.
CADx, however, can assess the prevalence or absence of disease, for example, or the severity, stage, and prognosis of disease. There are many other applications and examples of CADx software.
The FDA has the most experience with CADe software, and it has published guidances for 510(k) submissions and for clinical performance assessment, according to Sahiner.
De novo CADx ruling
In a significant development, the FDA in July accepted a de novo request of classification from image analysis software developer Quantitative Insights for its QuantX Advanced breast CADx software, which is based on artificial intelligence technology. The FDA concluded that the device, and substantially equivalent devices of this generic type, should be considered class II and therefore eligible for 510(k) premarket notification.
The acceptance of the de novo request established a new regulation -- 21 CFR 892.2060 -- for this new generic device type, which is defined as "Radiological computer-assisted diagnostic (CADx) software for lesions suspicious for cancer." The FDA said that this type of software characterizes lesions based on features or information extracted from the images and provides information about the lesion(s) to the user. Importantly, diagnostic and patient management decisions are still made by the clinical user, Sahiner said. The FDA's ruling does not specify a particular imaging modality or cancer type for this device.
"This is a path forward for similar devices for CADx," he said.
The FDA noted that 510(k) submissions for radiological CADx software for lesions suspicious for cancer must include the following:
- Device description
- Description of performance testing protocols and dataset used to assess whether device will improve reader performance
- Standalone performance testing protocols and results
- Results from performance testing protocols that demonstrate that the device improves reader performance
- Appropriate software documentation
These guidelines are similar to the FDA's CADe guidelines, according to Sahiner.
Continuously learning algorithms
Delving further into the issues involved with machine learning, Sahiner noted that many algorithms -- especially those that use deep learning -- are designed to learn from a training dataset how to perform a task without being explicitly programmed to do so. Other types of algorithms learn from experience, continuously learning over time.
"A continuously learning system, as opposed to a locked system that is locked until it's updated, can benefit public health by making use of ever-enlarging datasets for algorithm training, by adapting to the environment, by adapting to individual patients, or [by adapting to] different geographic or patient populations," Sahiner said.
A number of questions remain regarding continuously learning systems, such as how to ensure that updates do not compromise the safety and effectiveness of the device.
"How the users adapt to an evolving algorithm is another question," he said. "And if your device evolves by collecting new data, how [do you] control the quality of new training data? And when should the algorithm update occur? Each time you get a slightly enlarged dataset or when your performance -- however you measure it -- gets a jump? These are all technical questions that we should think about."
Another important question is whether performance testing should be conducted after each algorithm modification, Sahiner said. In addition, the reuse of datasets for testing algorithms remains an important concern.
"High-quality test datasets with reference truth are often limited in medicine, so there's a lot of temptation to use the same dataset over and over again when you're trying to assess the performance of the algorithm after each modification," he said. "But if you do it too many times, then your so-called 'test' set becomes either part of training or more of a validation dataset. So the performance on that dataset may seem to be improving, whereas your performance for the true population may not."
A possible mitigation strategy for test dataset reuse is thresholdout, a method that uses differential privacy techniques to avoid the problems that occur with reusing test datasets, according to Sahiner. The DIDSR is currently researching this topic.
Other initiatives
Sahiner also serves as co-leader of the Xavier Health Continuously Learning Systems Working Group, which launched in August at the Xavier Health AI Summit. The group brings together experts from medical device and pharmacology industries, academia, and government, Sahiner said.
"Our purpose is to try [to determine] what kind of information we need to provide a reasonable level of confidence in the performance of continuously learning systems," he said.
Sahiner also noted that the Center for Devices and Radiological Health has established a digital health unit, which announced in July a Digital Health Innovation Action Plan for addressing the unique aspects of digital health and software products. As part of an integrated approach for regulating these products, the FDA is exploring a new streamlined pathway for software. Under a pilot precertification program, the FDA will test a regulatory approach that provides streamlined software review for companies that demonstrate a culture of quality and organizational excellence.
"Based on the [software's] classification risk and [the company's] precertification level, the product may go immediately to commercial distribution or it might go to a streamlined premarket review," Sahiner said. "Then the real-world data collected could maybe feed back into this loop."
Nine selected companies will be participating in the pilot program, he said.