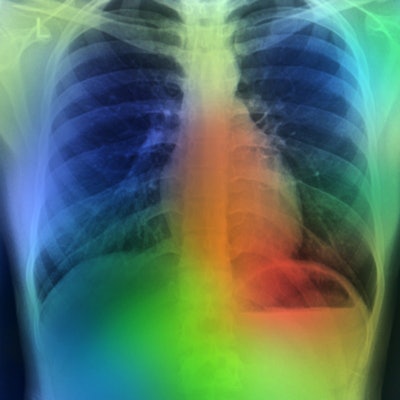
By analyzing chest radiographs and a patient's electronic medical record (EMR), a deep-learning algorithm was able to identify more high-risk smokers who could benefit from CT lung cancer screening than current Medicare eligibility criteria, according to research published September 1 in the Annals of Internal Medicine.
A team of researchers from Massachusetts General Hospital led by Dr. Michael Lu developed CXR-LC, a fusion convolutional neural network that can predict the risk of future lung cancer based on EMR data such as chest radiographs, age, sex, and smoking status. In testing, CXR-LC offered better predictions for long-term incident lung cancer than eligibility criteria from the U.S. Centers for Medicare and Medicaid Services (CMS) for CT lung cancer screening. It also yielded comparable results to a commonly used lung-cancer risk prediction model.
Risk model comparisons
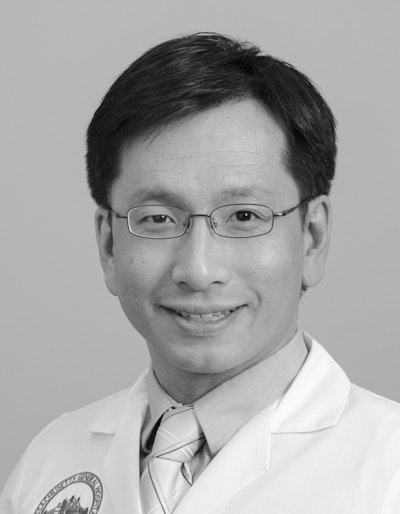
The researchers trained and fine-tuned CXR-LC using data from 41,856 subjects in the Prostate, Lung, Colorectal, and Ovarian (PLCO) Cancer Screening Trial. They then validated the model on an additional 5,615 smokers from the PLCO trial with 12 years of follow-up and an external dataset of 5,493 heavy smokers from the National Lung Screening Trial (NLST) with six years of follow-up.
Risk probabilities provided by CXR-LC were converted to an ordinal risk score: low (< 2%), indeterminate (2% to < 3.297%,), high (3.297% to < 8%), and very high ( ≥ 8%).
The researchers compared the algorithm's performance with that of CT lung cancer screening eligibility criteria from CMS and also PLCOM2012, a lung cancer logistic regression risk-prediction model with 11 types of data inputs.
Performance of CXR-LC for predicting patients with future incident lung cancer | ||||
CMS eligibility criteria for CT lung cancer screening | PLCOM2012 risk score | CXR-LC AI model | PLCOM2012 risk score and CXR-LC AI model | |
AUC on PLCO test et (12-year risk) | 0.634 | 0.761 | 0.755 | 0.790 |
AUC on NLST test set (6-year risk) | n/a | 0.650 | 0.659 | 0.686 |
CXR-LC statistically outperformed CMS eligibility criteria (p < 0.001) in the PLCO test set. What's more, the combination of CXR-LC and PLCOM2012 was better than PLCOM2012 alone in both the PLCO (p = 0.016) test set and the NLST test set (p = 0.010).
To provide a fair comparison among all of the risk models, the researchers also reviewed performance of the models at risk thresholds that yield the same screening population size as the CMS eligibility criteria. In that analysis, CXR-LC yielded 74.9% sensitivity on the PLCO dataset, compared with 74.4% from the PLCOM2012 risk score and 63.8% using CMS eligibility criteria. The difference between CXR-LC and the CMS eligibility criteria was statistically significant (p = 0.012). The algorithm missed 30.7% fewer incident lung cancers than the CMS criteria would have, according to the researchers.
After performing decision curve analysis, the authors also judged that CXR-LC had higher net benefit than CMS eligibility and similar benefit to PLCOM2012.
The researchers noted, however, that they would not recommend performing chest radiography on its own to assess lung cancer risk.
"Instead, a pragmatic future implementation of CXR-LC could analyze existing chest radiographs from outpatient smokers by using an automated EMR tool," they wrote.
CXR-LC can analyze a standard radiograph in less than half a second on a consumer-grade computer, according to the researchers. A high CXR-LC risk score could then trigger an EMR alert to perform a targeted interview to assess risk and discuss lung cancer screening.
"This 2-stage approach using CXR-LC as an automated EMR screen followed by a targeted risk and screening eligibility interview addresses the primary goal of getting more high-risk smokers into the screening pipeline while retaining the confidence of traditional risk scores," they wrote.