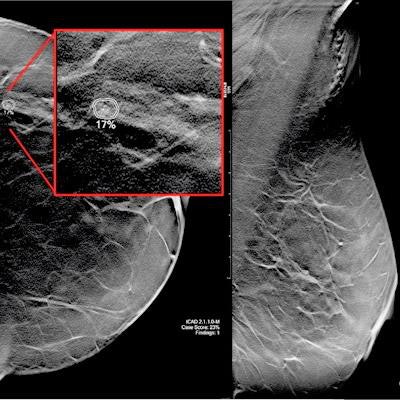
Artificial intelligence (AI) software may help reduce the workloads of breast imagers by helping them focus on the digital breast tomosynthesis (DBT) exams that are most likely to have cancer, according to research presented at ECR 2021.
A commercially available software application (ProFound AI, iCAD) allowed a research team led by Dr. Emily Conant, chief of breast imaging at the University of Pennsylvania, to identify one-third of screening DBT exams to triage and remove from priority review, with zero false negatives. When risk factors like breast density and age were added to the AI analysis, Conant et al found that more than half of cases could be triaged, with no false negatives.
The increasing use of DBT has improved the cancer detection rate and reduced recalls in breast screening. But because DBT machines also acquire larger datasets, they can require longer interpretation times. Conant's group wanted to determine if AI can triage this workload by indicating which cases were the least likely to have cancer.
"DBT improves screening by decreasing false positives and increasing cancer detection when combined with digital mammography," Conant asked. "Yet, we know that these tomosynthesis exams are associated with significantly longer interpretation times. Can we look with a deep-learning AI application and say there's a group of mammograms that just really aren't going to have cancer?"
So they performed a study to evaluate if ProFound AI could identify DBT exams at low likelihood of cancer and help triage workloads. Conant and colleagues started with consecutive cases from 18 U.S. and three French sites. They found a total of 506 cases with cancer and 1,293 noncancer cases. The dataset had a cancer detection rate of 6 cancers per 1,000 women screened.
The AI algorithm generated a case score that indicated the likelihood that a case included cancer; the researchers set the algorithm to operate with zero false negatives. They then measured the algorithm's impact on workload by calculating the percentage of cases that could be triaged based on its recommendation.
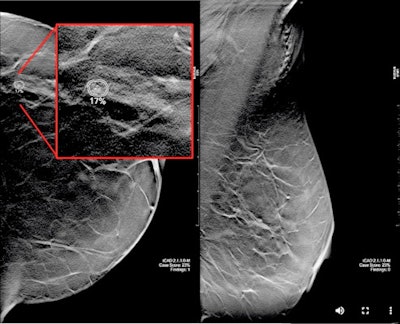
When it came to triaging cases, the algorithm selected 33.4% of exams that would be suitable for triage because they were unlikely to have cancer. When age and breast density were added to the case score, that number rose to 58.6%.
The question is, what then happens to the cases that are triaged? Conant was hesitant to state that they shouldn't be reviewed at all, but she did suggest that review could be deprioritized. For example, in Europe, it's standard practice to double read all mammograms. Perhaps AI could select DBT exams that are only read by one radiologist -- saving precious resources.
"If there is a way to take the completely negative cases out of a worklist, they could be read later or, some suggest that maybe someday, not read at all," Conant said in an email to AuntMinnie.com. "I'm not there yet myself!"
She noted that in the U.S., the triage concept could be applied to prioritize those mammograms that should be reviewed immediately. Or, AI could be used to quickly determine which women should be asked to stay in the radiology department after their mammogram to receive additional imaging -- saving them an extra trip.
"These case scores could potentially further refine who should get supplemental screening with, say, abbreviated breast MR," Conant said. "The cases with high scores -- with imaging measures beyond just subjective density alone -- to include the complexity of the parenchyma pattern and AI-CAD scores based on masses, calcifications, asymmetries across the two breasts, etc. could be triaged to have MR recommended."
Conant noted limitations included a relatively small number of cases, even though they did use four different vendors.
"There were no clinically discovered false negatives included in our dataset, and we certainly need to validate in a separate large data," she said.
She concluded by saying that DBT is becoming the "new" mammogram, but that long-term follow-up studies are needed to assess important clinical outcomes, such as the actual impact on morbidity and mortality of patients.
And what about AI?
"Well, it's here," she said. "I think we need to embrace it, and we will become better radiologists."