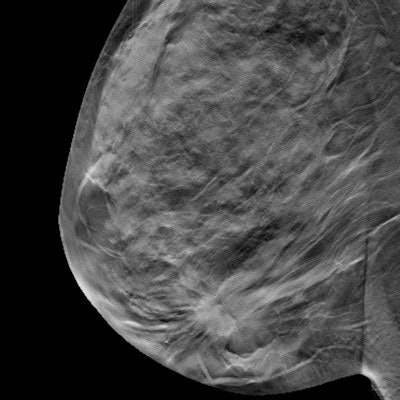
Digital breast tomosynthesis (DBT) has gone mainstream, and its benefits are legion compared with digital mammography. Adding artificial intelligence (AI) to DBT could boost its value even more, a group from NYU Langone Health suggests.
Using AI with DBT could not only streamline a radiology department's workflow but could also improve women's health, wrote a team led by Dr. Julia Goldberg of NYU Langone Health in New York City. The group's commentary was published November 4 in RadioGraphics.
"Further development of AI models for DBT has the potential to lead to improved practice efficiency and ultimately improved patient health outcomes of breast cancer screening and diagnostic evaluation," the authors noted.
Most AI applications for breast imaging have been developed for digital mammography, in part because there are some technical challenges for developing AI for DBT, such as the large datasets the technology generates, the complexity of its annotations, and smaller training sets.
Nonetheless, there are at least seven ways AI could increase DBT's benefits, according to Goldberg and colleagues.
- Improving cancer detection sensitivity/accuracy. "[Studies] have demonstrated that the use of AI algorithms results in noninferior or improved sensitivity for cancer detection compared with the sensitivity of traditional methods of reading screening DBT studies in the clinical setting," the team wrote.
- Decreasing the workload. "One approach to ameliorating the trend of increasing work volumes is to implement AI-based triage systems in which normal images are labeled nonmalignant and thus not interpreted by radiologists, while abnormal images are evaluated by radiologists," Goldberg and colleagues explained.
- Decreasing recall rates. "In a [study that performed a] clinical simulation of 13,306 DBT examinations ... the AI-identified low-suspicion DBT studies automatically excluded from radiologist review were not recalled," the authors noted.
- Localizing/classifying abnormal findings. "One common strategy for lesion localization is to train a convolutional neural network model to create bounding boxes around suspicious imaging findings ... each bounding box may be associated with a different confidence level for malignancy," the group wrote.
- Better visualization of suspicious lesions. "In computer-aided detection-based approaches, suspicious regions are identified on the DBT sections and then projected onto a 2D domain to render a synthesized digital mammography image with increased depictability," the authors explained.
- Lowering radiation doses: "Efforts are also underway to reduce the radiation dose of the tomosynthesis volume ... [and] ... noise, lower radiation doses, and improve image quality," they noted.
- Assessing breast cancer risk, especially in women with dense tissue. "AI models may further contribute to more accurate breast density estimation of suspicious lesions," the team wrote.
"At each step of the breast imaging process, from the decision to perform screening and image acquisition to the reporting of imaging findings and follow-up recommendations, there are multiple potential opportunities for AI to augment the benefits of DBT," Goldberg and colleagues concluded.