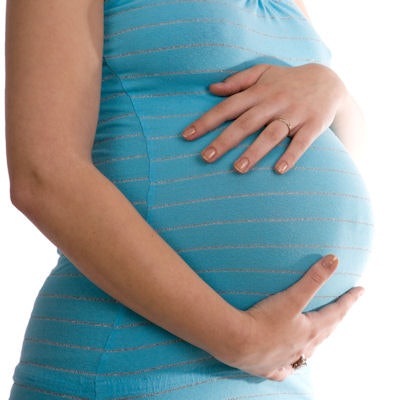
Making use of 3D MRI features, a machine-learning algorithm can accurately predict pregnancies with fetal growth restriction (FGR), potentially opening up opportunities for interventions to protect vulnerable fetuses, according to research published online in the Journal of Magnetic Resonance Imaging (July 22, 2017).
Researchers from Children's National Health System in Washington, DC, have developed a machine-learning framework that assesses placental shape and texture features on MR images. In testing, it was able to distinguish healthy pregnancies from FGR pregnancies with accuracy and specificity rates of more than 85% each. What's more, their method also performed well in estimating birth weight for both healthy and high-risk fetuses.
"This novel approach allows us to assess placental 'health and wellness' in vivo and is providing important insights into normal and abnormal placental development," said senior author Catherine Limperopoulos, PhD, co-director of research in the institution's division of neonatology. "This, in turn, can assist in accurately identifying fetuses who are not growing appropriately in utero, which can lead to the development of future therapeutic interventions to optimize fetal growth and development."
Assessing placental health
Placental insufficiency is a leading cause of fetal growth restriction and adverse outcomes, but reliable biomarkers for determining the point at which placental failure adversely affects the fetus and mother aren't presently available, according to Limperopoulos.
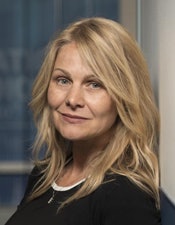
"There is a pronounced lack of information about normal placental development in utero and across gestation from which to reliably identify placental dysfunction," she told AuntMinnie.com.
The researchers targeted this problem by developing advanced MRI tools that go beyond the traditional MRI approach of lesion detection and enable noninvasive measurements of in vivo placental structure.
For their study, the researchers acquired 124 fetal scans from 80 pregnancies, beginning at the 18th week of gestation and continuing through the 39th week. Of the 80 women, 46 had normal pregnancies and healthy fetuses and 34 had pregnancies that were complicated by FGR -- estimated fetal weight below the 10th percentile for gestational age.
All single-shot, fast spin-echo, T2-weighted images were acquired on a 1.5-tesla Discovery MR450 system (GE Healthcare) with an eight-channel surface receiver coil and two sets of acquisition parameters. Placental volumes were then manually segmented on the images by an annotator with more than one year of experience in MRI placental segmentation. Next, 3D image "meshes" of these segmentations were reconstructed using a meshing framework developed by their institution based on the Computational Geometry Algorithms Library. Finally, a Poisson surface reconstruction was performed to obtain the final 3D surface that was used for analysis.
Placental shape and texture
The shape of the placenta on the MR study was then characterized using three 3D features: volume, thickness, and elongation. Placental texture was evaluated with three different sets of textural features computed on the entire placenta, according to the group. They then applied two machine-learning frameworks to predict FGR and birth rate. The framework for predicting FGR was based on the RUSBoost algorithm, while a machine-learning model based on regression forests was used to estimate birth weight.
The machine-learning method accurately predicted pregnancies with FGR:
- Accuracy: 86%
- Precision: 77%
- Recall: 86%
It also did reasonably well for predicting birth weight:
- Mean percentage error ± standard deviation (SD) for healthy fetuses: 0.3 ± 13.4%
- Mean percentage error ± SD for FGR: -2.6 ± 15.9%
Limperopoulos noted that placental dysfunction can result in fetal and neonatal death, preterm birth, FGR, and long-term neurodevelopmental morbidity.
"Current techniques, such as ultrasound, Doppler, and biophysical profile, cannot provide direct measures of placental [and fetal] well-being," she said. "At best, these techniques provide surrogate measures that lack sensitivity and specificity. The recent successful application of medical AI holds the promise of supporting the development of novel biomarkers to identify high-risk pregnancies."
Current status
The group is currently testing its methods on a larger clinical cohort using serial fetal MRI scans.
"We are developing novel quantitative MRI measures that characterize the complex and dynamic features underlying the development and evolution of placental morphology, microstructure, perfusion, and oxygen transport in healthy pregnancies across gestation," Limperopoulos said. "We have amassed a database from [over] 300 normal pregnancies of serial longitudinal placental MRI studies in the second and third trimester. These data form the substrate for our studies of normal placental structure and function across gestation."
The overarching goal is to identify key, in vivo quantitative MR developmental signatures from which to construct a multimodal model of normal placental development, she said.
"As part of the strategy for defining these developmental markers, we will compare these data with data previously obtained from women with known placental failure, which will allow us to define early, important deviations from normal in the high-risk pregnancy before placental dysfunction compromises fetal and maternal health," she said. "We are currently testing the ability for these biomarkers to detect early differences in placental structure and function in high-risk pregnancies.