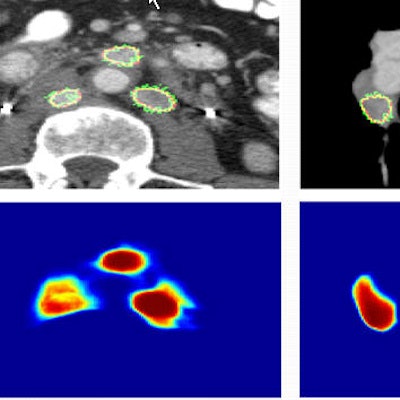
CHICAGO - A new automated lymph-node segmentation system applies both neural networks and structured optimization methods to the difficult task of separating lymph nodes from similar surrounding tissues, according to a presentation at the 2016 RSNA meeting.
The results could significantly improve cancer care by making lymph-node measurements more accurate, concluded researchers from the U.S. National Institutes of Health (NIH).
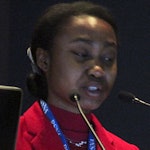
The group combined holistically nested neural networks (HNNs) and structured optimization techniques to solve the problem of lymph nodes that are barely visible on CT. The method actually trains two HNNs, built on convolutional neural networks and deeply supervised networks, to learn lymph-node cluster appearance (HNN-A) or contour (HNN-C) probabilistic output maps from thoracoabdominal CT images.
These measures are components in the recently developed concept of boundary neural fields (BNF), which matched up the ground truth lesion measurements with more than 80% accuracy.
"We designed an automatic segmentation method for thoracoabdominal lymph-node clusters ... with integration of HNN learning and structured optimization," said presenter Isabella Nogues. Boundary neural fields were the most accurate segmentation method, she said.
Hard to measure
"Thoracoabdominal lesions are among the most challenging to analyze on CT," Nogues said. "The reason for this is that CT images of this region display very poor intensity and texture contrast. This is due to the fact that lymph nodes are very similar in intensity to many of the surrounding tissues."
Also, the high frequency of lymph-node clusters and the ambiguous boundaries of lymph nodes make accurate measurement very difficult, she said.
The objective of the study by Nogues, Dr. Ronald Summers, PhD, and colleagues was twofold: First, the researchers wanted to design a fully automated segmentation method for thoracoabdominal lymph-node clusters. Next, they wanted to measure the volume of the clusters as a more accurate estimation than diameter, typically measured using Response Evaluation Criteria in Solid Tumors (RECIST).
The study population included 84 abdominal and 87 mediastinal patients from the NIH Clinical Center. In all, they generated 16,268 axial slices from 3D thoracoabdominal CT, acquired during the portal-venous phase with 1- to 1.25-mm slice thicknesses. There were 395 abdominal and 295 mediastinal lymph nodes in the publicly available dataset.
HNN first generates class label maps with the same resolution as the input image. Then, HNN-A and HNN-C predictions are formulated into unary and pairwise terms of conditional random fields (CRFs). The CRFs are subsequently solved using three standardized output methods: dense CRF (dCRF), graph cuts (GC), and the recently developed boundary neural fields. All of these segmentation predictions are used to compute lymph-node cluster volumes on the dataset.
An expert radiologist segmented all enlarged lymph nodes with short-axis diameters of at least 10 mm (volume range, 0.24-31.74 cc; mean, 11.75 ± 25.05 cc).
Boundary problem solved
Boundary neural fields delivered the highest quantitative results, with a mean Dice coefficient between segmented and ground truth lymph-node volumes of 82.1% ± 9.6%, compared with 73% ± 17.6% for HNN-A, 69% ± 22% for dense CRFs, and 67.3% ± 16.8% for graph cuts.
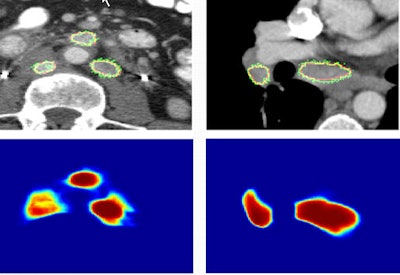
A paired t-test comparing ground truth with segmented lymph-node volumes produced the following p-values: 0.87 for BNF, 0.37 for HNN-A, 0.10 for dCRF, and less than 0.01 for GC, the authors wrote in an abstract.
"The main conclusion was that boundary neural field was the most accurate segmentation method, which is very promising for the development of imaging biomarkers based on volume measurements," Nogues said. "These volume measurements, in turn, could potentially improve RECIST measurements for lymph nodes."