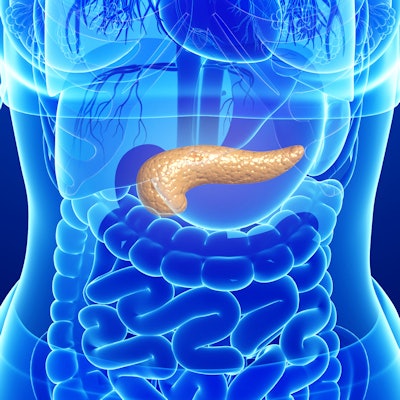
Deep-learning algorithms can be used to provide automated and accurate segmentation of the pancreas on CT exams, according to research presented at the recent Society for Imaging Informatics in Medicine (SIIM) annual meeting.
A team of researchers from the Mayo Clinic in Rochester, MN, has trained a model that yields highly accurate segmentations of the pancreas. Importantly, the algorithm performed as well on normal cases as it did with pancreatic ductal adenocarcinoma cases, according to presenter Panagiotis Korfiatis, PhD,
Estimated pancreatic volume on CT can be used as a biomarker in assessing a number of diseases, including diabetes. Furthermore, segmentation of the pancreas is required for artificial intelligence (AI)-based cancer detection models. Unfortunately, manual segmentation of the pancreas is time-consuming and cumbersome, and intra- and inter-reader variability is unknown, according to the researchers.
For AI models to be used clinically, the process has to be automated in order to provide a fast, scalable implementation that's independent of variation between users, Korfiatis said. However, pancreas segmentation is a challenging task.
"The pancreas is a small organ with considerable variability and complexity in anatomy, location, shape, and attenuation," he said. "In addition, the tumor can be isodense with the pancreas or the peripancreatic tissue, which further enhances the difficulty of segmenting the ... pancreas."
As part of an effort to develop software for early detection of pancreatic cancer, the researchers sought to utilize a 3D convolutional neural network to automatically segment the pancreas. Their deep-learning algorithm operates in two phases. After first placing a bounding box around the pancreas on the CT image, the model then segments the pancreas and places the segmentation in the original CT dataset.
The researchers trained, validated, and tested their algorithm on an internal dataset of 2,639 abdominal CT scans acquired during the portal-venous phase between 2006 and 2017. These exams were acquired on scanners from multiple vendors at a slice thickness of ≤ 5 mm.
The CT studies included 519 cases of pancreatic ductal adenocarcinoma. Of these, 1,612 were used for training and 284 were utilized for validation. The algorithm was then tested on the remaining 473 exams, which included 110 pancreatic ductal adenocarcinoma cases.
After internal testing, the authors then tested the algorithm on two external datasets: the Medical Segmentation Decathlon dataset (102 cases of pancreatic ductal adenocarcinoma) and the U.S. National Institutes of Health (NIH) Pancreas-CT dataset (80 normal cases). To provide the ground truth for the study, three radiologists performed volumetric pancreas segmentation using the Nvidia organ segmentation mode on version 4.11 of the 3D Slicer software.
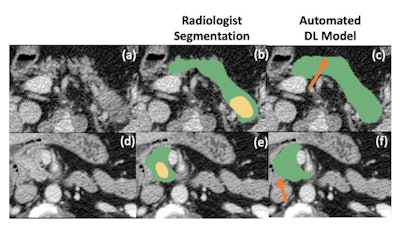
Performance of deep-learning algorithm for pancreas segmentation | |||
Internal test set | NIH Pancreas-CT dataset (normal cases) | Medical Segmentation Decathlon dataset (cancer cases) | |
Mean Dice similarity coefficient | 0.892 | 0.855 | 0.865 |
The model's segmentation performance did not differ significantly between normal and pancreatic ductal adenocarcinoma cases, Korfiatis said.
"Our analyses also revealed that the ground-truth segmentations in the NIH-PCT dataset tended to miss minor parts of the pancreas in certain cases, which were, in fact, included in the model-predicted segmentations," he said.
Also, the Dice similarity coefficient produced by the algorithm in cancer cases is similar to the level of interobserver variability -- Dice similarity coefficient of 0.85 -- shown in a previous study, according to Korfiatis.
He noted that the automated segmentation errors were commonly seen at the interface of the pancreatic head and duodenum, as well as the pancreatic tail.
"These regions of the pancreas are very difficult to delineate in some cases, even for an expert radiologist and this further contributes to the larger intra-reader and inter-reader variability that can be observed for this task," Korfiatis said.