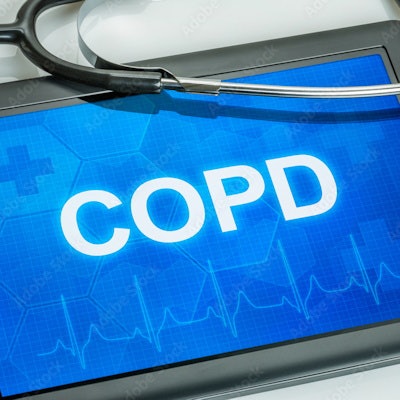
Radiomic features on CT such as parenchymal texture and lung and airway shape can help clinicians accurately identify chronic obstructive pulmonary disease (COPD), a study published June 20 in Radiology has found.
The findings underscore CT's promise for its opportunistic use for this indication, according to a team led by Praneeth Puchakayala of the University of Alabama in Birmingham.
"With the increasing use of low-dose CT for lung cancer screening, there is also a need for an accurate algorithm for detecting COPD," the group wrote.
COPD is the third leading cause of death around the world -- in the U.S., there are 12 million adults who suffer with it -- and half of these cases are undiagnosed, the team noted. Chest CT is a common exam for many indications and offers an opportunity to detect the disease; add machine learning to the modality and it becomes an even more powerful tool for diagnosing COPD.
"[It] is pertinent to note that approximately 70 million chest CT scans are acquired each year in the United States," the group noted. "These scans present opportunities to detect COPD in previously undiagnosed individuals."
Puchakayala and colleagues investigated whether there are particular CT radiomic features that could flag COPD; they used data from the Genetic Epidemiology of COPD study, or COPDGene, to conduct research that included 8,878 patients, all of which underwent both standard dose (visit 1) and low-dose (visit 3) CT exams.
The team assessed results from patients' baseline exams and exams performed 10 years later, evaluating demographics, CT emphysema percentage, radiomics features, and a combined feature set that used information from inspiratory CT exams alone. The group used two AI algorithms -- one for the baseline CT exam and one for the low-dose CT exam -- and assessed the performance on test sets using the area under the receiver operating characteristic curve (AUC) measure.
AUC values for detecting COPD using AI algorithms on standard and low-dose CT | ||||
Feature | Model 1 (standard CT) | p-value | Model 2 (low-dose CT) | p-value |
Radiomics | 0.9 | Reference | 0.87 | Reference |
Demographics | 0.73 | < 0.001 | 0.0 | 0.001 |
Emphysema percentage | 0.82 | < 0.001 | 0.74 | 0.002 |
Combined features | 0.9 | 0.16 | 0.88 | 0.32 |
"[We] showed that the morphologic (shape) features of lung and airways contribute most to COPD detection on low-dose CT images, whereas CT density and textural features contribute significantly to COPD detection on standard-dose CT images," the group reported.
There's more research to be done, according to Puchakayala and colleagues.
"More studies are needed to test these algorithms in a nonsmoking cohort, as well as in the presence of coexisting lung diseases," they concluded.
The complete study can be found here.